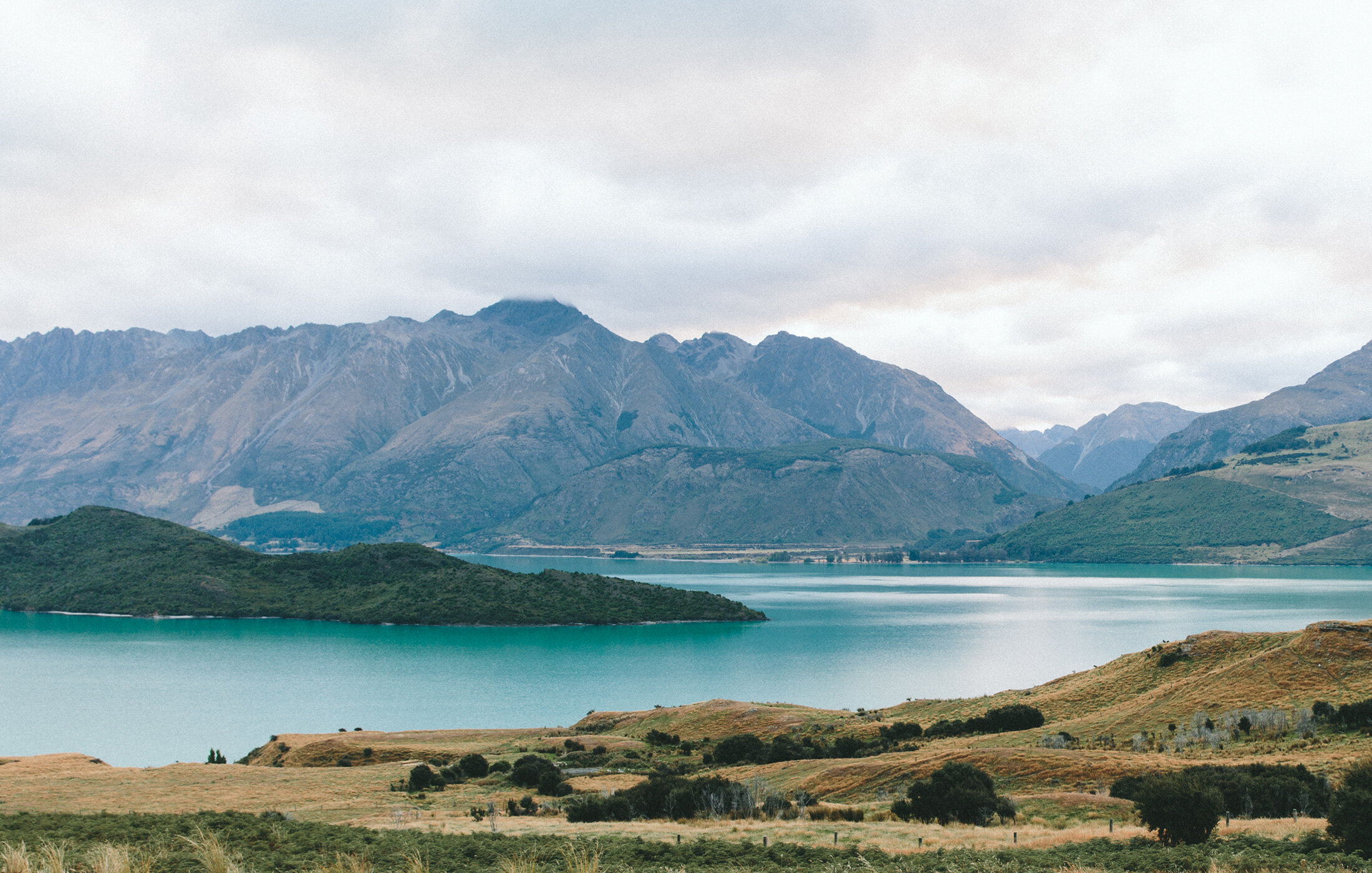
Papers on eQTLs, gene regulatory networks, statistical algorithms and applications to several diseases
-
Large-scale cis-and trans-eQTL analyses identify thousands of genetic loci and polygenic scores that regulate blood gene expression. Võsa U, Claringbould A, …, Esko T, Franke L. Nat Genet. 2021
In this paper we show that through an integrated eQTL approach we can identify the downstream consequences for many disease associated genetic variants on networks of genes.
-
Brain expression quantitative trait locus and network analysis reveals downstream effects and putative drivers for brain-related diseases. De Klein, …, Franke L, Westra HJ. Nat Genet. 2023
In this study we integrated large-scale brain eQTL data and conducted network analysis to identify genes that could be putative drivers of different neurodegenerative diseases.
-
Single-cell RNA sequencing identifies celltype-specific cis-eQTLs and co-expression QTLs. van der Wijst MGP, Brugge H, de Vries DH, Deelen P, Swertz MA; LifeLines Cohort Study; BIOS Consortium, Franke L. Nat Genet. 2018
In this paper we described how genetic variation affects single-cell gene expression levels and observed that genetic variants can have strong effects on the regulatory wiring of cells. By constructing personalized co-expression networks, we observed striking differences between individuals.
-
Identification of context-dependent expression quantitative trait loci in whole blood. Zhernakova DV, Deelen P, Vermaat M, van Iterson M, … 't Hoen PA, Franke L. Nat Genet. 2017
Here we observed that genetic variants have strong effects on gene-expression-levels that are highly context-specific. We then used this phenomenon to identify upstream regulators of downstream gene-expression-levels, paving the way for the reconstruction of directed regulatory networks.
-
Biological interpretation of genome-wide association studies using predicted gene functions. Pers TH, Karjalainen JM, Chan Y, … Hirschhorn JN, Franke L. Nat Commun. 2015
Here we used a reconstructed gene co-expression network, based on 77,840 gene expression profiles, to predict gene functions. We subsequently showed that these predicted functions permitted improved biological interpretation of GWAS, leading to our DEPICT method.
-
Improving the diagnostic yield of exome- sequencing by predicting gene–phenotype associations using large-scale gene expression analysis. Deelen P, …, Franke L. Nat Commun 2019
We reanalysed 31,499 RNA-seq samples to make a gene regulatory network (GeneNetwork.nl), while also predicting disease symptoms for mutated genes, enabling us to identify new genes that cause Mendelian diseases.
-
Gene expression analysis identifies global gene dosage sensitivity in cancer. Fehrmann RS, Karjalainen JM, Krajewska M, … , van Vugt MA, Franke L. Nat Genet. 2015
We studied ~80,000 gene expression profiles and studied the effect of somatic copy number alterations on gene-expression-levels, and observed that 96% of all genes are dosage-sensitive. We identified a large number of physiological components that can be computationally be corrected for.
-
Systematic identification of trans eQTLs as putative drivers of known disease associations. Westra HJ, Peters MJ, Esko T, … van Meurs JBJ, Franke L. Nat Genet. 2013
In this paper we studied the effect of genetic variation on gene-expression-levels in 5,311 samples, which enabled us to identify the effects on gene expression of many genetic variants associated with disease.